CoviDetector: A transfer learning-based semi supervised approach to detect Covid-19 using CXR images
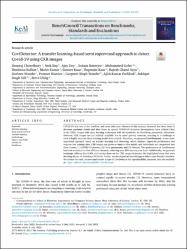
Göster/ Aç
Erişim
info:eu-repo/semantics/openAccessTarih
2023Yazar
Chowdhury, DeeprajDas, Anik
Dey, Ajoy
Banerjee, Soham
Golec, Muhammed
Kollias, Dimitrios
Kumar, Mohit
Kaur, Guneet
Kaur, Rupinder
Arya, Rajesh Chand
Wander, Gurleen
Wander, Praneet
Wander, Gurpreet Singh
Parlikad, Ajith Kumar
Gill, Sukhpal Singh
Uhlig, Steve
Üst veri
Tüm öğe kaydını gösterÖzet
COVID-19 was one of the deadliest and most infectious illnesses of this century. Research has been done to
decrease pandemic deaths and slow down its spread. COVID-19 detection investigations have utilised Chest
X-ray (CXR) images with deep learning techniques with its sensitivity in identifying pneumonic alterations.
However, CXR images are not publicly available due to users’ privacy concerns, resulting in a challenge to
train a highly accurate deep learning model from scratch. Therefore, we proposed CoviDetector, a new semisupervised approach based on transfer learning and clustering, which displays improved performance and
requires less training data. CXR images are given as input to this model, and individuals are categorised into
three classes: (1) COVID-19 positive; (2) Viral pneumonia; and (3) Normal. The performance of CoviDetector
has been evaluated on four different datasets, achieving over 99% accuracy on them. Additionally, we generate
heatmaps utilising Grad-CAM and overlay them on the CXR images to present the highlighted areas that were
deciding factors in detecting COVID-19. Finally, we developed an Android app to offer a user-friendly interface.
We release the code, datasets and results’ scripts of CoviDetector for reproducibility purposes; they are available
at: https://github.com/dasanik2001/CoviDetector